PEMBELAJARAN MESIN UNTUK MENILAI KELAYAKAN KREDIT PROYEK RETROFIT: MULTINOMIAL LOGIT
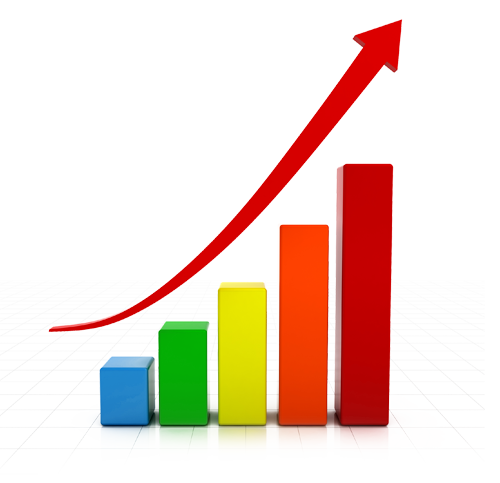
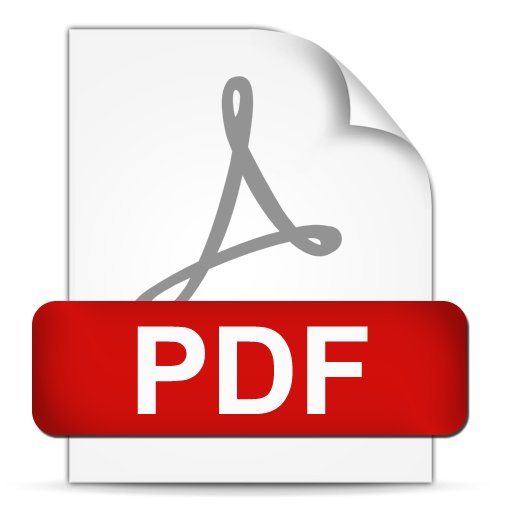
Abstract
Creditworthiness assessment was one of the first areas to apply machine learning techniques in economics. The creditworthiness of retrofit protection was vital for ESCO in determining the credit scoring. This study aimed to develop a retrofitting assessment model to utilize machine learning with multinomial logistic (MNL) and life cycle cost analysis (LCCA). This study aims to provide an evaluation of creditworthiness models from the evaluation of financing alternative in Indonesia's energy efficiency industry. The goal was to reduce the total of prediction error, which comprised bias, variance, and fundamental error. The findings demonstrated that machine learning approaches might yield significantly greater prediction accuracy. In addition, machine learning is also expected to automatically capture the nonlinear relationship between input features and selected results. This study is also expected to draw on ideas from machine learning to develop an enhanced model for retrofitting creditworthiness research and suggest new research directions.
Downloads
References
Almeida, A. De, Santos, B., Paolo, B., & Quicheron, M. (2014). Solid state lighting review – Potential and challenges in Europe. Renewable and Sustainable Energy Reviews, 34, 30–48.
Athey, S. (2017). The Impact of Machine Learning on Economics, (September), 1–27.
Athey, S., & Imbens, G. W. (2019). Machine Learning Methods Economists Should Know About. Sloan Foundation.
Ben-Akiva, M., & Boccara, B. (1995). Discrete choice models with latent choice sets. International Journal of Research in Marketing, 12(1), 9–24.
Bennich, P. (2015). Test Report – Clear, Non-Directional LED Lamps. Branson, W. H. (1979). Macroeconomic Theory And Policy.
Breiman, L. (2001). Random Forests.
Cherchi, E., & Cirillo, C. (2010). Day-to-day variability and habit in modal choices: a mixed logit model on panel data. In XVI PANAM (pp. 1–19). Portugal.
Christopher, M., Belghith, A., Weinreb, R. N., Bowd, C., Goldbaum, M. H., Saunders, L. J., … Zangwill, L. M. (2018). Retinal nerve fiber layer features identified by unsupervised machine learning on optical coherence tomography scans predict glaucoma progression. Investigative Ophthalmology and Visual Science, 59(7), 2748–2756.
Cramer, J. A. (2004). A Systematic Review of Adherence With Medications for Diabetes. Diabetes Care, 27(5), 1218–1224.
Dobbs, R., Pohl, H., Lin, D.-Y., Mischke, J., Garemo, N., Hexter, J., … Nanavatty, R. (2013). Infrastructure productivity: How to save $1 trillion a year.
Dupont, L., Fliche, O., & Yang, S. (2020). Governance of Artificial Intelligence in Finance. Retrieved from https://acpr.banque-france.fr/sites/default/files/medias/documents/20200612_ai_governance_finance.pdf
Fornara, F., Pattitoni, P., Mura, M., & Strazzera, E. (2016). Predicting intention to improve household energy efficiency: The role of value-belief-norm theory, normative and informational influence, and specific attitude. Journal of Environmental Psychology, 45, 1–10.
Frankel, D., Heck, S., & Tai, H. (2013). Sizing the potential of behavioral energy-efficiency initiatives in the US residential market. McKinsey&Company.
Fuller, S. K., & Petersen, S. R. (1996). LCCosting Manual for the Federal Energy Management Program. NIST Handbook 135.
Grennepois, N., Alvirescu, M., and Bombail, M. (2018). Using random forest for credit risk models. Deloitte Risk Advisory, September, 2018.
Heesen, F., & Madlener, R. (2016). Technology Acceptance as Part of the Energy Performance Gap in Energy- Efficient Retrofitted Dwellings. Institute for Future Energy Consumer Needs and Behavior (FCN).
Hejazi, F. A., Ramanathan, R. A., & Jaffar, M. S. (2016). Seismic Response of a Light Rail Transit Station Equipped with Braced Viscous Damper. Science & Technology, 24(2), 273–283.
Hensher, D. A., & Greene, W. H. (2002). The mixed logit model: The state of practice. Working Paper ITS-WP-02-01 (Vol. 30).
Husin, N. I., Ahmad, A. C., Wahid, A. M. A., & Kamaruzzaman, S. N. (2017). Energy Efficiency Criteria for Green Highway. Social Science & Humanities, 25, 119–128.
Johnstone, M. L., & Tan, L. P. (2014). Exploring the Gap Between Consumers’ Green Rhetoric and Purchasing Behaviour. Journal of Business Ethics, 132(2), 311–328.
Lessmann, S., Baesens, B., Seow, H. V., & Thomas, L. C. (2015). Benchmarking state-of-the-art classification algorithms for credit scoring: An update of research. European Journal of Operational Research, 247(1), 124–136.
Liang, X., Peng, Y., & Shen, G. Q. (2016). A game theory based analysis of decision making for green retrofit under different occupancy types. Journal of Cleaner Production, 137, 1300–1312.
McWilliams, J. A., & Walker, I. S. (2005). Home Energy Article : A Systems Approach to Retrofitting Residential HVAC Systems. Lawrence Berkeley National Laboratory.
Mearig, T., Coffee, N., & Morgan, M. (1999). Life Cycle Cost Analysis Handbook.
Mullainathan, S., & Spiess, J. (2017). Machine learning: An applied econometric approach. Journal of Economic Perspectives, 31(2), 87–106.
Polzin, F., von Flotow, P., & Nolden, C. (2016). Modes of governance for municipal energy efficiency services - The case of LED street lighting in Germany. Journal of Cleaner Production, 139, 133– 145.
Ruparathna, R., Hewage, K., & Sadiq, R. (2017). Economic evaluation of building energy retrofits: A fuzzy based approach. Energy and Buildings.
Stern, P. C. (2011). Contributions of Psychology to Limiting Climate Change. American Psychologist, 66(4), 303–314.
US Environmental Protection Agency. (2008). ENERGY STAR - Building Upgrade Manual.
Varian, H. R. (2014). Big data: New tricks for econometrics. Journal of Economic Perspectives, 28(2), 3–28.
West, D. (2000). Neural network credit scoring models. Computers and Operations Research, 27(11– 12), 1131–1152.
Wiginton, J. C. (1980). University of Washington School of Business Administration Cambridge University Press. Journal of Financial and Quantitative Analysis, XV(3), 757–770.
Yobas, M. B., Crook, J. N., & Ross, P. (2000). Credit scoring using neural and evolutionary techniques. IMA Journal of Mathematics Applied in Business and Industry, 11(2), 111–125.

This work is licensed under a Creative Commons Attribution-ShareAlike 4.0 International License.
- Copyright on articles is retained by the respective author(s), without restrictions. A non-exclusive license is granted to Akuntansi dan Teknologi Informasi (JATI) to publish the article and identify itself as its original publisher, along with the commercial right to include the article in a hardcopy issue for sale to libraries and individuals.
- Articles published in Akuntansi dan Teknologi Informasi (JATI) are licensed under a Creative Commons Attribution-ShareAlike 4.0 International license. You are free to copy, transform, or redistribute articles for any lawful purpose in any medium, provided you give appropriate credit to the original author(s) and the journal, link to the license, indicate if changes were made, and redistribute any derivative work under the same license.
- By publishing in Akuntansi dan Teknologi Informasi (JATI), authors grant any third party the right to use their article to the extent provided by the Creative Commons Attribution-ShareAlike 4.0 International license.