Sentiment Analysis for Sumber Gempong Rice Field-Based Tourism Destination using Long Short-Term Memory
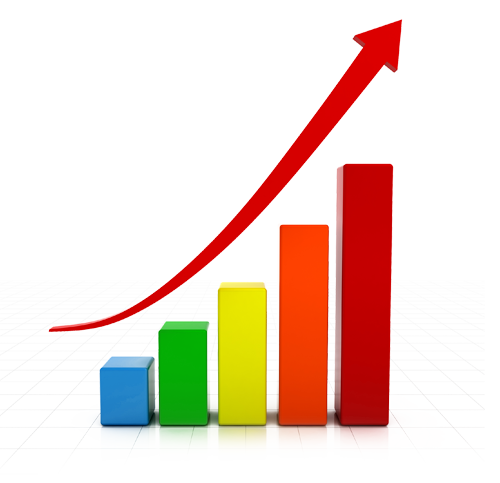
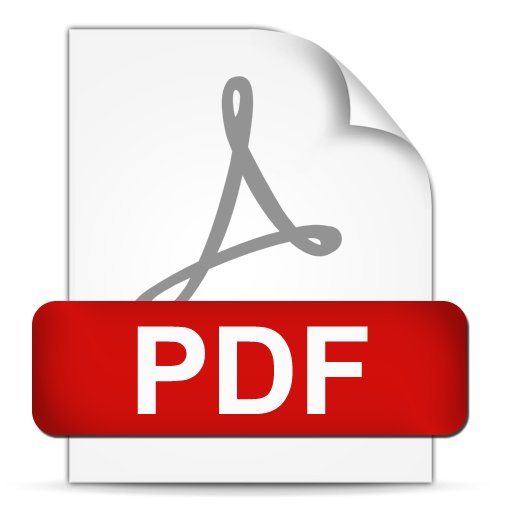
Abstract
Abstract—Sumber Gempong is a rice field-based tourist destination located in Ketapanrame Village, Trawas District, Mojokerto Regency, East Java Province. It is managed by a village-owned company (BUMDesa Mutiara Welirang). BUMDesa evaluates tourist satisfaction manually by reviewing online comments and it consumes time and labor works. Data used in this research automatically collected from Google Maps Review. Long Short-Term Memory (LSTM) method analyze data of two sentiment labels, positive or negative, based on four categories: facilities, services, culinary, and attractions. The collected dataset has 674 comments consist of 420 positive sentiments and 254 negative sentiments with 320 facilities, 61 services, 125 culinary, and 192 attractions comments. Five LSTM models were trained on each of four categories and an overall category. The trained models of overall, facilities, services, culinary, and attractions categories achieved, respectively, 91.2%, 86.8%, 94.1%, 89.7%, and 95.6% of accuracies. The average result accuracy is 91.48%. The manager of BUMDesa Mutiara Welirang satisfied with the results of the system and the sentiment results can be used as evaluation material for Sumber Gempong.
Keywords: sentiment anaylsis, LSTM, deep learning, social media, tourism
Abstrak—Wisata Sawah Sumber Gempong berada di Desa Ketapanrame, Kecamatan Trawas, Kabupaten Mojokerto dan merupakan tempat wisata alam yang dikelola oleh BUMDesa Mutiara Welirang. Evaluasi terhadap tempat wisata ini dilakukan dengan membaca secara manual ulasan-ulasan yang ditulis di media sosial dan pengamatan pribadi. Banyaknya jumlah ulasan yang ada menjadi kendala dalam melakukan evaluasi karena membutuhkan waktu yang cukup lama. Penelitian ini mengambil data ulasan secara otomatis dari media sosial yang diberi label positif atau negatif berdasarkan empat kategori, yaitu fasilitas, pelayanan, kuliner, dan wahana. Metode Long Short-Term Memory (LSTM) dipakai sebagai alat untuk melakukan analisis sentimen. Pengambilan data secara otomatis mendapatkan 674 ulasan yang dibagi menjadi 420 ulasan positif dan 254 ulasan negatif, dengan 320 ulasan fasilitas, 61 ulasan pelayanan, 125 ulasan kuliner , dan 192 ulasan wahana. Lima buah model dilatih berdasar tiap kategorinya dan kategori secara keseluruhan. Model yang telah dilatih mendapatkan nilai akurasi sebesar 91,2%, 86,8%, 94,1%, 89,7%, dan 95,6% berturut-turut untuk keseluruhan kategori, kategori fasilitas, layanan, kuliner, dan wahana. Rata-rata akurasi mencapai 91,48%. Hasil dari sistem telah diujicobakan kepada manajer BUMDesa Mutiara Welirang dan bisa dipakai sebagai bahan evalusi untuk peningkatan kualitas di Sumber Gempong.
Kata kunci: analisis sentimen, LSTM, deep learning, media sosial, wisata
Downloads
References
Ahn, H. and Park, E., 2023. Motivations for user satisfaction of mobile fitness applications: An analysis of user experience based on online review comments. Humanities and Social Sciences Communications, 10(1). https://doi.org/10.1057/s41599-022-01452-6.
Alita, D. and Isnain, A.R., 2020. Pendeteksian Sarkasme pada Proses Analisis Sentimen Menggunakan Random Forest Classifier. jurnal komputasi, 8(2). https://doi.org/10.23960/komputasi.v8i2.2615.
Arsi, P. and Waluyo, R., 2021. Analisis Sentimen Wacana Pemindahan Ibu Kota Indonesia Menggunakan Algoritma Support Vector Machine (SVM). Jurnal Teknologi Informasi dan Ilmu Komputer, 8(1), p.147. https://doi.org/10.25126/jtiik.0813944.
Azzahra, S.A. and Wibowo, A., 2020. Analisis Sentimen Multi-Aspek Berbasis Konversi Ikon Emosi dengan Algoritme Naïve Bayes untuk Ulasan Wisata Kuliner Pada Web Tripadvisor. Jurnal Teknologi Informasi dan Ilmu Komputer, 7(4), p.737. https://doi.org/10.25126/jtiik.2020731907.
Benrouba, F. and Boudour, R., 2023. Emotional sentiment analysis of social media content for mental health safety. Social Network Analysis and Mining, 13(1). https://doi.org/10.1007/s13278-022-01000-9.
Borrego, Á. and Comalat Navarra, M., 2020. What users say about public libraries: an analysis of Google Maps reviews. Online Information Review, 45(1), pp.84–98. https://doi.org/10.1108/OIR-09-2019-0291.
Budge, K., 2017. Objects in Focus: Museum Visitors and Instagram. Curator: The Museum Journal, 60(1), pp.67–85. https://doi.org/10.1111/cura.12183.
Caldo, D., Bologna, S., Conte, L., Amin, M.S., Anselma, L., Basile, V., Hossain, M.M., Mazzei, A., Heritier, P., Ferracini, R., Kon, E. and De Nunzio, G., 2023. Machine learning algorithms distinguish discrete digital emotional fingerprints for web pages related to back pain. Scientific Reports, 13(1). https://doi.org/10.1038/s41598-023-31741-2.
Disbudporapar, 2023. Data Wisatawan Desember 2022. Dinas Kebudayaan, Kepemudaan, Olahraga dan Pariwisata, Pemerintah Kabupaten Mojokerto.
Fu, M. and Pan, L., 2022. Sentiment Analysis of Tourist Scenic Spots Internet Comments Based on LSTM. Mathematical Problems in Engineering, 2022, pp.1–9. https://doi.org/10.1155/2022/5944954.
Fu, X., Liu, X. and Li, Z., 2024. Catching eyes of social media wanderers: How pictorial and textual cues in visitor-generated content shape users’ cognitive-affective psychology. Tourism Management, 100, p.104815. https://doi.org/10.1016/j.tourman.2023.104815.
Ginantra, N.L.W.S.R., Yanti, C.P., Prasetya, G.D., Sarasvananda, I.B.G. and Wiguna, I.K.A.G., 2022. Analisis Sentimen Ulasan Villa di Ubud Menggunakan Metode Naive Bayes, Decision Tree, dan K-NN. Jurnal Nasional Pendidikan Teknik Informatika : JANAPATI, [online] 11(3), pp.205–215. https://doi.org/10.23887/janapati.v11i3.49450.
Hanifah, R. and Nurhasanah, I.S., 2018. Implementasi Web Crawling untuk Mengumpulkan Informasi Wisata Kuliner di Bandar Lampung. Jurnal Teknologi Informasi dan Ilmu Komputer, 5(5), p.531. https://doi.org/10.25126/jtiik.201855842.
Hendriyani, I.G.A.D., 2023. Siaran Pers: Menparekraf: ADWI 2023 Perkuat Konsistensi Masyarakat Bangun Desa Wisata. [online] Kementerian Pariwisata dan Ekonomi Kreatif Republik Indonesia. Available at: <https://kemenparekraf.go.id/hasil-pencarian/siaran-pers-menparekraf-adwi-2023-perkuat-konsistensi-masyarakat-bangun-desa-wisata>.
Jassim, M.A., Abd, D.H. and Omri, M.N., 2023. Machine learning-based new approach to films review. Social Network Analysis and Mining, 13(1). https://doi.org/10.1007/s13278-023-01042-7.
Kaur, G. and Sharma, A., 2023. A deep learning-based model using hybrid feature extraction approach for consumer sentiment analysis. Journal of Big Data, 10(1). https://doi.org/10.1186/s40537-022-00680-6.
Khairunnisa, S., Adiwijaya, A. and Faraby, S. Al, 2021. Pengaruh Text Preprocessing terhadap Analisis Sentimen Komentar Masyarakat pada Media Sosial Twitter (Studi Kasus Pandemi COVID-19). JURNAL MEDIA INFORMATIKA BUDIDARMA, 5(2), p.406. https://doi.org/10.30865/mib.v5i2.2835.
Liliana, D.Y., Hikmah, N.N. and Harjono, M., 2021. Pengembangan Sistem Pemantauan Sentimen Berita Berbahasa Indonesia Berdasarkan Konten dengan Long-Short-Term Memory. Jurnal Teknologi Informasi dan Ilmu Komputer, 8(5), p.995. https://doi.org/10.25126/jtiik.2021854624.
Liu, X., Shi, T., Zhou, G., Liu, M., Yin, Z., Yin, L. and Zheng, W., 2023. Emotion classification for short texts: an improved multi-label method. Humanities and Social Sciences Communications, 10(1). https://doi.org/10.1057/s41599-023-01816-6.
Mahardika, F.R., Supianto, A.A., Setiawan, N.Y., Yuwana, R.S. and Suryawati, E., 2022. Rekomendasi Pengembangan Fasilitas Wisata Tugu Pahlawan Surabaya Melalui Visualisasi Dashboard Hasil Klasifikasi Analisis Sentimen Ulasan Pengunjung. Jurnal Teknologi Informasi dan Ilmu Komputer, 9(2), p.363. https://doi.org/10.25126/jtiik.2022925655.
Mathayomchan, B. and Taecharungroj, V., 2020. “How was your meal?” Examining customer experience using Google maps reviews. International Journal of Hospitality Management, 90, p.102641. https://doi.org/10.1016/j.ijhm.2020.102641.
Maulana, A.R., Wijoyo, S.H. and Mursityo, Y.T., 2023. Analisis Sentimen Kebijakan Penerapan Kurikulum Merdeka Sekolah Dasar dan Sekolah Menengah pada Media Sosial Twitter dengan Menggunakan Metode Word Embedding dan Long Short Term Memory Networks (LSTM). Jurnal Teknologi Informasi dan Ilmu Komputer, 10(3), p.523. https://doi.org/10.25126/jtiik.20231036977.
Mohammad, S., Bravo-Marquez, F., Salameh, M. and Kiritchenko, S., 2018. SemEval-2018 Task 1: Affect in Tweets. In: Proceedings of the 12th International Workshop on Semantic Evaluation. [online] New Orleans, Louisiana: Association for Computational Linguistics. pp.1–17. https://doi.org/10.18653/v1/S18-1001.
Mohammed, A. and Kora, R., 2019. Deep learning approaches for Arabic sentiment analysis. Social Network Analysis and Mining, 9(1), p.52. https://doi.org/10.1007/s13278-019-0596-4.
Negara, A.B.P., Muhardi, H. and Putri, I.M., 2020. Analisis Sentimen Maskapai Penerbangan Menggunakan Metode Naive Bayes dan Seleksi Fitur Information Gain. Jurnal Teknologi Informasi dan Ilmu Komputer, 7(3), p.599. https://doi.org/10.25126/jtiik.2020711947.
Ningrum, A.A., Syarif, I., Gunawan, A.I., Satriyanto, E. and Muchtar, R., 2021. Algoritma Deep Learning-LSTM untuk Memprediksi Umur Transformator. Jurnal Teknologi Informasi dan Ilmu Komputer, 8(3), p.539. https://doi.org/10.25126/jtiik.2021834587.
Normawati, D. and Prayogi, S.A., 2021. Implementasi Naïve Bayes Classifier Dan Confusion Matrix Pada Analisis Sentimen Berbasis Teks Pada Twitter. Jurnal Sains Komputer dan Informatika (J-SAKTI), 5(2).
Nurvania, J., Jondri, J. and Lhaksamana, K.M., 2021. Analisis Sentimen Pada Ulasan di TripAdvisor Menggunakan Metode Long Short-Term Memory (LSTM). eProceedings of Engineering, 8(4).
Oktaviana, N.E., Sari, Y.A. and Indriati, I., 2022. Analisis Sentimen terhadap Kebijakan Kuliah Daring Selama Pandemi Menggunakan Pendekatan Lexicon Based Features dan Support Vector Machine. Jurnal Teknologi Informasi dan Ilmu Komputer, 9(2), p.357. https://doi.org/10.25126/jtiik.2022925625.
Ombabi, A.H., Ouarda, W. and Alimi, A.M., 2020. Deep learning CNN–LSTM framework for Arabic sentiment analysis using textual information shared in social networks. Social Network Analysis and Mining, 10(1), p.53. https://doi.org/10.1007/s13278-020-00668-1.
Putra, R.P., Pratomo, A.H. and Perwira, R.I., 2022. Text Message Classification using Multiclass Support Vector Machine on Information Service Chatbot in the Informatics Department UPN “Veteran” Yogyakarta. Telematika, 19(3), p.295. https://doi.org/10.31315/telematika.v19i3.7418.
Qaqish, E., Aranki, A. and Etaiwi, W., 2023. Sentiment analysis and emotion detection of post-COVID educational Tweets: Jordan case. Social Network Analysis and Mining, 13(1). https://doi.org/10.1007/s13278-023-01041-8.
Samah, K.A.F.A., Azharludin, N.M.N., Riza, L.S., Jono, M.N.H.H. and Moketar, N.A., 2023. Classification and visualization: Twitter sentiment analysis of Malaysia’s private hospitals. IAES International Journal of Artificial Intelligence, 12(4), pp.1793–1802. https://doi.org/10.11591/ijai.v12.i4.pp1793-1802.
Saptari, R., 2021. Analisis Sentimen Pengguna Twitter Terhadap Pelayanan Unit Gawat Darurat Rumah Sakit Umum di Indonesia Menggunakan Seleksi Fitur Information Gain dan Support Vector Machine. Joined Journal (Journal of Informatics Education), 4(2), p.104. https://doi.org/10.31331/joined.v4i2.1925.
Selle, N., Yudistira, N. and Dewi, C., 2022. Perbandingan Prediksi Penggunaan Listrik dengan Menggunakan Metode Long Short Term Memory (LSTM) dan Recurrent Neural Network (RNN). Jurnal Teknologi Informasi dan Ilmu Komputer, 9(1), p.155. https://doi.org/10.25126/jtiik.2022915585.
Talaat, A.S., 2023. Sentiment analysis classification system using hybrid BERT models. Journal of Big Data, 10(1). https://doi.org/10.1186/s40537-023-00781-w.
Tunca, S., Sezen, B. and Wilk, V., 2023. An exploratory content and sentiment analysis of the guardian metaverse articles using leximancer and natural language processing. Journal of Big Data, 10(1). https://doi.org/10.1186/s40537-023-00773-w.
Wang, Y., Chu, C. and Lan, T., 2022. Sentiment Classification of Educational Tourism Reviews Based on Parallel CNN and LSTM with Attention Mechanism. Mobile Information Systems, 2022, pp.1–13. https://doi.org/10.1155/2022/6177427.
Yuliska, Y., Qudsi, D.H., Lubis, J.H., Syaliman, K.U. and Najwa, N.F., 2021. Analisis Sentimen pada Data Saran Mahasiswa Terhadap Kinerja Departemen di Perguruan Tinggi Menggunakan Convolutional Neural Network. Jurnal Teknologi Informasi dan Ilmu Komputer, 8(5), p.1067. https://doi.org/10.25126/jtiik.2021854842.
Yulita, W., 2021. Analisis Sentimen Terhadap Opini Masyarakat Tentang Vaksin Covid-19 Menggunakan Algoritma Naïve Bayes Classifier. Jurnal Data Mining dan Sistem Informasi, 2(2), p.1. https://doi.org/10.33365/jdmsi.v2i2.1344.

This work is licensed under a Creative Commons Attribution-ShareAlike 4.0 International License.
- Articles published in Keluwih: Jurnal Sains dan Teknologi are licensed under a Creative Commons Attribution-ShareAlike 4.0 International license. You are free to copy, transform, or redistribute articles for any lawful purpose in any medium, provided you give appropriate credit to the original author(s) and the journal, link to the license, indicate if changes were made, and redistribute any derivative work under the same license.
- Copyright on articles is retained by the respective author(s), without restrictions. A non-exclusive license is granted to Keluwih: Jurnal Sains dan Teknologi to publish the article and identify itself as its original publisher, along with the commercial right to include the article in a hardcopy issue for sale to libraries and individuals.
- By publishing in Keluwih: Jurnal Sains dan Teknologi, authors grant any third party the right to use their article to the extent provided by the Creative Commons Attribution-ShareAlike 4.0 International license.